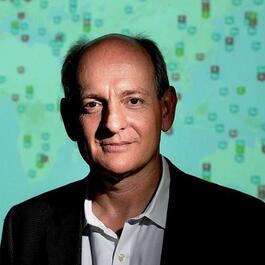
#80 – Stuart Russell on why our approach to AI is broken and how to fix it
Stuart Russell, Professor at UC Berkeley and co-author of the most popular AI textbook, thinks the way we approach machine learning today is fundamentally flawed. In his new book, Human Compatible, he outlines the 'standard model' of AI development, in which intelligence is measured as the ability to achieve some definite, completely-known objective that we've stated explicitly. This is so obvious it almost doesn't even seem like a design choice, but it is. Unfortunately there's a big problem with this approach: it's incredibly hard to say exactly what you want. AI today lacks common sense, and simply does whatever we've asked it to. That's true even if the goal isn't what we really want, or the methods it's choosing are ones we would never accept. We already see AIs misbehaving for this reason. Stuart points to the example of YouTube's recommender algorithm, which reportedly nudged users towards extreme political views because that made it easier to keep them on the site. This isn't something we wanted, but it helped achieve the algorithm's objective: maximise viewing time. Like King Midas, who asked to be able to turn everything into gold but ended up unable to eat, we get too much of what we've asked for. Links to learn more, summary and full transcript. This 'alignment' problem will get more and more severe as machine learning is embedded in more and more places: recommending us news, operating power grids, deciding prison sentences, doing surgery, and fighting wars. If we're ever to hand over much of the economy to thinking machines, we can't count on ourselves correctly saying exactly what we want the AI to do every time. Stuart isn't just dissatisfied with the current model though, he has a specific solution. According to him we need to redesign AI around 3 principles: 1. The AI system's objective is to achieve what humans want. 2. But the system isn't sure what we want. 3. And it figures out what we want by observing our behaviour. Stuart thinks this design architecture, if implemented, would be a big step forward towards reliably beneficial AI. For instance, a machine built on these principles would be happy to be turned off if that's what its owner thought was best, while one built on the standard model should resist being turned off because being deactivated prevents it from achieving its goal. As Stuart says, "you can't fetch the coffee if you're dead." These principles lend themselves towards machines that are modest and cautious, and check in when they aren't confident they're truly achieving what we want. We've made progress toward putting these principles into practice, but the remaining engineering problems are substantial. Among other things, the resulting AIs need to be able to interpret what people really mean to say based on the context of a situation. And they need to guess when we've rejected an option because we've considered it and decided it's a bad idea, and when we simply haven't thought about it at all. Stuart thinks all of these problems are surmountable, if we put in the work. The harder problems may end up being social and political. When each of us can have an AI of our own — one smarter than any person — how do we resolve conflicts between people and their AI agents? And if AIs end up doing most work that people do today, how can humans avoid becoming enfeebled, like lazy children tended to by machines, but not intellectually developed enough to know what they really want? Chapters:Rob’s intro (00:00:00)The interview begins (00:19:06)Human Compatible: Artificial Intelligence and the Problem of Control (00:21:27)Principles for Beneficial Machines (00:29:25)AI moral rights (00:33:05)Humble machines (00:39:35)Learning to predict human preferences (00:45:55)Animals and AI (00:49:33)Enfeeblement problem (00:58:21)Counterarguments (01:07:09)Orthogonality thesis (01:24:25)Intelligence explosion (01:29:15)Policy ideas (01:38:39)What most needs to be done (01:50:14) Producer: Keiran Harris. Audio mastering: Ben Cordell. Transcriptions: Zakee Ulhaq.
From "80,000 Hours Podcast"
Comments
Add comment Feedback