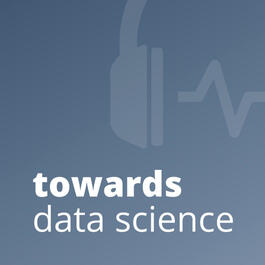
59. Matthew Stewart - Tiny ML and the future of on-device AI
When it comes to machine learning, we’re often led to believe that bigger is better. It’s now pretty clear that all else being equal, more data, more compute, and larger models add up to give more performance and more generalization power. And cutting edge language models have been growing at an alarming rate — by up to 10X each year. But size isn’t everything. While larger models are certainly more capable, they can’t be used in all contexts: take, for example, the case of a cell phone or a small drone, where on-device memory and processing power just isn’t enough to accommodate giant neural networks or huge amounts of data. The art of doing machine learning on small devices with significant power and memory constraints is pretty new, and it’s now known as “tiny ML”. Tiny ML unlocks an awful lot of exciting applications, but also raises a number of safety and ethical questions. And that’s why I wanted to sit down with Matthew Stewart, a Harvard PhD researcher focused on applying tiny ML to environmental monitoring. Matthew has worked with many of the world’s top tiny ML researchers, and our conversation focused on the possibilities and potential risks associated with this promising new field.
From "Towards Data Science"
Comments
Add comment Feedback