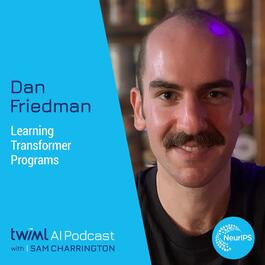
Learning Transformer Programs with Dan Friedman
Today, we continue our NeurIPS series with Dan Friedman, a PhD student in the Princeton NLP group. In our conversation, we explore his research on mechanistic interpretability for transformer models, specifically his paper, Learning Transformer Programs. The LTP paper proposes modifications to the transformer architecture which allow transformer models to be easily converted into human-readable programs, making them inherently interpretable. In our conversation, we compare the approach proposed by this research with prior approaches to understanding the models and their shortcomings. We also dig into the approach’s function and scale limitations and constraints. The complete show notes for this episode can be found at twimlai.com/go/667.
From "The TWIML AI Podcast (formerly This Week in Machine Learning & Artificial Intelligence)"
Comments
Add comment Feedback