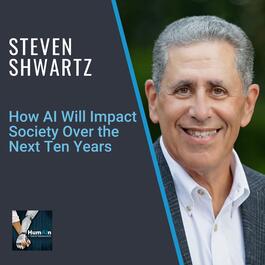
Steven Shwartz: How AI Will Impact Society Over the Next Ten Years
[Audio] Podcast: Play in new window | Download Subscribe: Google Podcasts | Spotify | Stitcher | TuneIn | RSS Steve received his PhD from Johns Hopkins University in Cognitive Science where he began his AI research and also taught Statistics at Towson State University. After receiving his PhD in 1979, AI pioneer Roger Schank invited Steve to join the Yale University faculty as a postdoctoral researcher in Computer Science. In 1981, Roger asked Steve to help him start one of the first AI companies, Cognitive Systems, which progressed to a public offering in 1986. Steve then started Esperant, which produced one of the leading Business Intelligence products of the 1990s. During the 1980s, Steve published 35 articles and a book on AI, spoke at many AI conferences, and received two commercial patents on AI. As the AI Winter of the 1990s set in, Steve transitioned into a career as a successful serial software entrepreneur and investor and created several companies that were either acquired or had a public offering. He tries to use his unique perspective as an early AI researcher and statistician to both explain how AI works in simple terms, to explain why people should not worry about intelligent robots taking over the world, and to explain the steps we need to take as a society to minimize the negative impacts of AI and maximize the positive impacts. Please support this podcast by checking out our sponsors: Episode Links: Steven Shwartz LinkedIn: https://www.linkedin.com/in/steveshwartz/ Steven Shwartz Twitter: https://twitter.com/sshwartz Steven Shwartz Website: https://www.device42.com Podcast Details: Podcast website: https://www.humainpodcast.com Apple Podcasts: https://podcasts.apple.com/us/podcast/humain-podcast-artificial-intelligence-data-science/id1452117009 Spotify: https://open.spotify.com/show/6tXysq5TzHXvttWtJhmRpS RSS: https://feeds.redcircle.com/99113f24-2bd1-4332-8cd0-32e0556c8bc9 YouTube Full Episodes: https://www.youtube.com/channel/UCxvclFvpPvFM9_RxcNg1rag YouTube Clips: https://www.youtube.com/channel/UCxvclFvpPvFM9_RxcNg1rag/videos Support and Social Media: – Check out the sponsors above, it’s the best way to support this podcast – Support on Patreon: https://www.patreon.com/humain/creators – Twitter: https://twitter.com/dyakobovitch – Instagram: https://www.instagram.com/humainpodcast/ – LinkedIn: https://www.linkedin.com/in/davidyakobovitch/ – Facebook: https://www.facebook.com/HumainPodcast/ – HumAIn Website Articles: https://www.humainpodcast.com/blog/ Outline: Here’s the timestamps for the episode: (00:00) – Introduction (09:42) – So most of the things that are taking jobs for example, is conventional software, not AI software. (10:57)- Exactly. And that's automated but it's conventional software. It's not AI. And most of the examples of where computers are replacing people, it's conventional software. It's not AI software. (14:49)- How you get data quality into your AI models and it's what they do that's really interesting. And I hadn't actually focused on it until I talked to this company. There's a big industry to clean data for tools like business intelligence that have been around for a long time. And there are, there are companies that are multi-billion dollar companies that provide data, cleaning tools, data extraction, and so forth. (17:13)- Everybody thought that with AI, you could diagnose illnesses from medical images better than the radiologists. And it's never actually worked out that way. I have friends who are radiologists, who use those AI tools and they say yes, sometimes they find things that I might've missed. But at the same time, they miss things that we would have found. (22:17)- I think we're seeing a lot of the rollout of a specific type of AI supervised learning, which is a type of machine learning. We're seeing it applied in many different areas. I actually have a database I keep before every time I see a new application of supervised learning and it's fascinating. It's being used in almost every area of business, of government, of the nonprofit world. It is fascinating how much application there is. (27:06)- And they're not really going to make sense if you drill down into them. So what's going to be the implication of that. Is it only going to be useful if there's all kinds of search engine optimization where you don't really care If what you're right makes sense. We're going to generate a lot of crap using GPT three and put it out there for search engine optimization purposes. (31:19)- And I think there's a lot of opportunity for companies that are helping develop software and services to help companies build non-biased explainable systems. And then you have a whole issue around when you build a machine learning system, it deteriorates over time. So it might only work for a couple of days and then start to go downhill. It might work for weeks, but you have to monitor those systems and go back and retrain them when the performance goes down. And all of that is a lot of effort. Advertising Inquiries: https://redcircle.com/brands Privacy & Opt-Out: https://redcircle.com/privacy
From "HumAIn Podcast"
Comments
Add comment Feedback