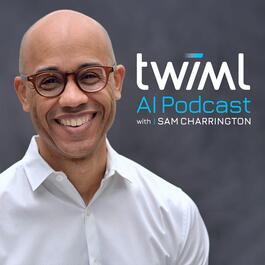
Dynamic Token Merging for Efficient Byte-level Language Models with Julie Kallini
Today, we're joined by Julie Kallini, PhD student at Stanford University to discuss her recent papers, “MrT5: Dynamic Token Merging for Efficient Byte-level Language Models” and “Mission: Impossible Language Models.” For the MrT5 paper, we explore the importance and failings of tokenization in large language models—including inefficient compression rates for under-resourced languages—and dig into byte-level modeling as an alternative. We discuss the architecture of MrT5, its ability to learn language-specific compression rates, its performance on multilingual benchmarks and character-level manipulation tasks, and its performance and efficiency. For the “Mission: Impossible Language Models” paper, we review the core idea behind the research, the definition and creation of impossible languages, the creation of impossible language training datasets, and explore the bias of language model architectures towards natural language. The complete show notes for this episode can be found at https://twimlai.com/go/724.
From "The TWIML AI Podcast (formerly This Week in Machine Learning & Artificial Intelligence)"
Comments
Add comment Feedback