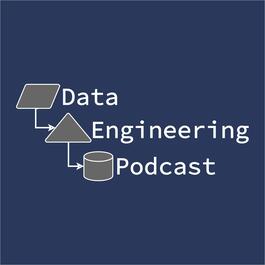
Pachyderm with Daniel Whitenack - Episode 1
Summary Do you wish that you could track the changes in your data the same way that you track the changes in your code? Pachyderm is a platform for building a data lake with a versioned file system. It also lets you use whatever languages you want to run your analysis with its container based task graph. This week Daniel Whitenack shares the story of how the project got started, how it works under the covers, and how you can get started using it today! Preamble Hello and welcome to the Data Engineering Podcast, the show about modern data infrastructure Go to dataengineeringpodcast.com to subscribe to the show, sign up for the newsletter, read the show notes, and get in touch. You can help support the show by checking out the Patreon page which is linked from the site. To help other people find the show you can leave a review on iTunes, or Google Play Music, and tell your friends and co-workers Your host is Tobias Macey and today I’m interviewing Daniel Whitenack about Pachyderm, a modern container based system for building and analyzing a versioned data lake. Interview with Daniel Whitenack Introduction How did you get started in the data engineering space? What is pachyderm and what problem were you trying to solve when the project was started? Where does the name come from? What are some of the competing projects in the space and what features does Pachyderm offer that would convince someone to choose it over the other options? Because of the fact that the analysis code and the data that it acts on are all versioned together it allows for tracking the provenance of the end result. Why is this such an important capability in the context of data engineering and analytics? What does Pachyderm use for the distribution and scaling mechanism of the file system? Given that you can version your data and track all of the modifications made to it in a manner that allows for traversal of those changesets, how much additional storage is necessary over and above the original capacity needed for the raw data? For a typical use of Pachyderm would someone keep all of the revisions in perpetuity or are the changesets primarily just useful in the context of an analysis workflow? Given that the state of the data is calculated by applying the diffs in sequence what impact does that have on processing speed and what are some of the ways of mitigating that? Another compelling feature of Pachyderm is the fact that it natively supports the use of any language for interacting with your data. Why is this such an important capability and why is it more difficult with alternative solutions? How did you implement this feature so that it would be maintainable and easy to implement for end users? Given that the intent of using containers is for encapsulating the analysis code from experimentation through to production, it seems that there is the potential for the implementations to run into problems as they scale. What are some things that users should be aware of to help mitigate this? The data pipeline and dependency graph tooling is a useful addition to the combination of file system and processing interface. Does that preclude any requirement for external tools such as Luigi or Airflow? I see that the docs mention using the map reduce pattern for analyzing the data in Pachyderm. Does it support other approaches such as streaming or tools like Apache Drill? What are some of the most interesting deployments and uses of Pachyderm that you have seen? What are some of the areas that you are looking for help from the community and are there any particular issues that the listeners can check out to get started with the project? Keep in touch Daniel Twitter – @dwhitena Pachyderm Website Free Weekend Project GopherNotes Links AirBnB RethinkDB Flocker Infinite Project Git LFS Luigi Airflow Kafka Kubernetes Rkt SciKit Learn Docker Minikube General Fusion The intro and outro music is from The Hug by The Freak Fandango Orchestra / CC BY-SA
From "Data Engineering Podcast"
Comments
Add comment Feedback