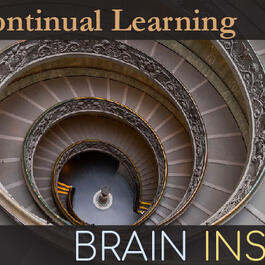
BI 123 Irina Rish: Continual Learning
Support the show to get full episodes, full archive, and join the Discord community. Irina is a faculty member at MILA-Quebec AI Institute and a professor at Université de Montréal. She has worked from both ends of the neuroscience/AI interface, using AI for neuroscience applications, and using neural principles to help improve AI. We discuss her work on biologically-plausible alternatives to back-propagation, using "auxiliary variables" in addition to the normal connection weight updates. We also discuss the world of lifelong learning, which seeks to train networks in an online manner to improve on any tasks as they are introduced. Catastrophic forgetting is an obstacle in modern deep learning, where a network forgets old tasks when it is trained on new tasks. Lifelong learning strategies, like continual learning, transfer learning, and meta-learning seek to overcome catastrophic forgetting, and we talk about some of the inspirations from neuroscience being used to help lifelong learning in networks. Irina's website.Twitter: @irinarishRelated papers:Beyond Backprop: Online Alternating Minimization with Auxiliary Variables.Towards Continual Reinforcement Learning: A Review and Perspectives.Lifelong learning video tutorial: DLRL Summer School 2021 - Lifelong Learning - Irina Rish. 0:00 - Intro 3:26 - AI for Neuro, Neuro for AI 14:59 - Utility of philosophy 20:51 - Artificial general intelligence 24:34 - Back-propagation alternatives 35:10 - Inductive bias vs. scaling generic architectures 45:51 - Continual learning 59:54 - Neuro-inspired continual learning 1:06:57 - Learning trajectoriesSupport the show to get full episodes, full archive, and join the Discord community. Irina is a faculty member at MILA-Quebec AI Institute and a professor at Université de Montréal. She has worked from both ends of the neuroscience/AI interface, using AI for neuroscience applications, and using neural principles to help improve AI. We discuss her work on biologically-plausible alternatives to back-propagation, using "auxiliary variables" in addition to the normal connection weight updates. We also discuss the world of lifelong learning, which seeks to train networks in an online manner to improve on any tasks as they are introduced. Catastrophic forgetting is an obstacle in modern deep learning, where a network forgets old tasks when it is trained on new tasks. Lifelong learning strategies, like continual learning, transfer learning, and meta-learning seek to overcome catastrophic forgetting, and we talk about some of the inspirations from neuroscience being used to help life
From "Brain Inspired"
Comments
Add comment Feedback