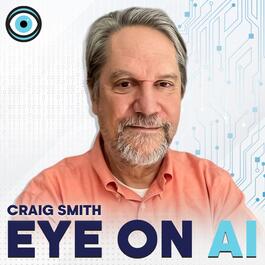
#247 Barr Moses: Why Reliable Data is Key to Building Good AI Systems
This episode is sponsored by Netsuite by Oracle, the number one cloud financial system, streamlining accounting, financial management, inventory, HR, and more. NetSuite is offering a one-of-a-kind flexible financing program. Head to https://netsuite.com/EYEONAI to know more. In this episode of Eye on AI, Craig Smith sits down with Barr Moses, Co-Founder & CEO of Monte Carlo, the pioneer of data and AI observability. Together, they explore the hidden force behind every great AI system: reliable, trustworthy data. With AI adoption soaring across industries, companies now face a critical question: Can we trust the data feeding our models? Barr unpacks why data quality is more important than ever, how observability helps detect and resolve data issues, and why clean data—not access to GPT or Claude—is the real competitive moat in AI today. What You’ll Learn in This Episode: Why access to AI models is no longer a competitive advantage How Monte Carlo helps teams monitor complex data estates in real-time The dangers of “data hallucinations” and how to prevent them Real-world examples of data failures and their impact on AI outputs The difference between data observability and explainability Why legacy methods of data review no longer work in an AI-first world Stay Updated: Craig Smith on X:https://x.com/craigss Eye on A.I. on X: https://x.com/EyeOn_AI (00:00) Intro (01:08) How Monte Carlo Fixed Broken Data (03:08) What Is Data & AI Observability? (05:00) Structured vs Unstructured Data Monitoring (08:48) How Monte Carlo Integrates Across Data Stacks (13:35) Why Clean Data Is the New Competitive Advantage (16:57) How Monte Carlo Uses AI Internally (19:20) 4 Failure Points: Data, Systems, Code, Models (23:08) Can Observability Detect Bias in Data? (26:15) Why Data Quality Needs a Modern Definition (29:22) Explosion of Data Tools & Monte Carlo’s 50+ Integrations (33:18) Data Observability vs Explainability (36:18) Human Evaluation vs Automated Monitoring (39:23) What Monte Carlo Looks Like for Users (46:03) How Fast Can You Deploy Monte Carlo? (51:56) Why Manual Data Checks No Longer Work (53:26) The Future of AI Depends on Trustworthy Data
From "Eye On A.I."
Comments
Add comment Feedback